Welcome to our comprehensive lesson on two-variable data analysis for the SAT! This crucial topic appears frequently on the exam and tests your ability to understand relationships between different variables. In this lesson, we'll explore how to interpret scatterplots, understand correlation, and work with linear models. By the end, you'll be able to confidently tackle any two-variable data problem on the SAT, even if you're starting with no prior knowledge.
Mastering Two-Variable Data: Models and Scatterplots for the SAT
Introduction
What are Two-variable data: models and scatterplots?
Two-variable data analysis involves examining the relationship between two different variables. For example, we might look at how study time relates to test scores, or how temperature affects ice cream sales.
A scatterplot is a graphical representation of two-variable data. Each point on a scatterplot represents one data pair (x, y), where x is the independent variable (plotted on the horizontal axis) and y is the dependent variable (plotted on the vertical axis).
Models are mathematical equations that describe the relationship between the variables. On the SAT, you'll primarily work with linear models, which have the form
where:
- is the slope (rate of change)
- is the y-intercept (value of y when x = 0)
The SAT tests your ability to interpret scatterplots, identify patterns in data, determine correlation, and use or create models to make predictions.
Free Full Length SAT Tests withOfficial-StyleQuestions
Practice with our full length adapive full testreal test-likequestions and proven300+points score boost
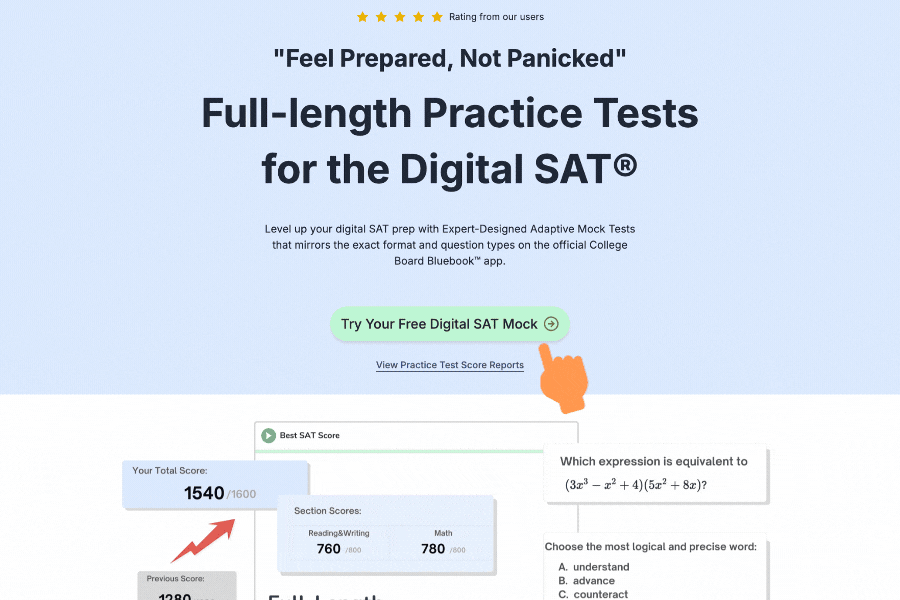
How to Use Two-variable data: models and scatterplots
Reading and Interpreting Scatterplots
-
Identify the variables: Always note what's being measured on each axis.
-
Observe the pattern: Look for trends in the data points.
- Positive correlation: As x increases, y tends to increase (points trend upward from left to right)
- Negative correlation: As x increases, y tends to decrease (points trend downward from left to right)
- No correlation: No clear pattern between x and y (points scattered randomly)
-
Assess the strength of correlation:
- Strong correlation: Points closely follow a pattern
- Weak correlation: Points follow a pattern but with significant scatter
Working with Linear Models
-
Finding the line of best fit:
- The line of best fit (regression line) is a linear model that best represents the trend in the data
- On the SAT, you'll often be given this equation or asked to interpret it
-
Interpreting the slope:
- The slope represents the rate of change in y per unit change in x
- Example: If the model is , then y increases by 3 units for each 1-unit increase in x
-
Interpreting the y-intercept:
- The y-intercept is the value of y when x = 0
- Example: In , when x = 0, y = 5
-
Making predictions:
- Substitute a value of x into the model to predict the corresponding y-value
- Be careful not to extrapolate too far beyond the given data range
-
Residuals:
- A residual is the difference between an actual y-value and the predicted y-value from the model
- Residual = Actual y-value - Predicted y-value
- Positive residuals are points above the line; negative residuals are points below the line
Two-variable data: models and scatterplots Worksheet
Practice Worksheet
Imagine a scatterplot showing the relationship between hours studied (x) and test scores (y) for 10 students:
Hours Studied (x) | Test Score (y) |
---|---|
1 | 65 |
2 | 70 |
3 | 75 |
4 | 78 |
5 | 82 |
6 | 85 |
7 | 89 |
8 | 92 |
9 | 94 |
10 | 96 |
Tasks:
-
Describe the correlation shown in this data (direction and strength).
-
The linear model for this data is approximately . Interpret the meaning of the slope and y-intercept in context.
-
Use the model to predict the test score for a student who studies 12 hours.
-
Calculate the residual for the student who studied 5 hours.
-
If a student scored 88 after studying, how many hours did they likely study according to the model?
Answers:
-
The data shows a strong positive correlation. As hours studied increases, test scores consistently increase.
-
Slope (3.5): For each additional hour studied, the test score is expected to increase by 3.5 points.
Y-intercept (62): A student who studies 0 hours is predicted to score 62 points on the test. -
For x = 12: . However, since the maximum score might be 100, this prediction may not be realistic (demonstrates the danger of extrapolation).
-
Actual y-value = 82. Predicted y-value = .
Residual = 82 - 79.5 = 2.5. The actual score is 2.5 points higher than predicted. -
If y = 88: → → hours (approximately 7 hours and 26 minutes).
Two-variable data: models and scatterplots Examples
Example 1
Example 1: Interpreting a Scatterplot
A researcher collected data on the number of hours 15 students spent on social media per day (x) and their GPA (y). The scatterplot shows a moderate negative correlation.
Question: What does this pattern suggest about the relationship between social media use and GPA?
Solution: The moderate negative correlation suggests that as the number of hours spent on social media increases, GPA tends to decrease. However, since the correlation is moderate (not strong), other factors likely influence GPA as well. We cannot conclude that social media use causes lower GPAs, only that there's an association between the variables.
Example 2
Example 2: Interpreting a Linear Model
A linear model relating the distance (d) in miles from a city center to the average home price (p) in thousands of dollars is given by: .
Question: Interpret the meaning of the slope and y-intercept in this context.
Solution:
- The slope (-15) indicates that for each additional mile away from the city center, the average home price decreases by $15,000.
- The y-intercept (350) represents the average home price at the city center (when d = 0), which is $350,000.
Example 3
Example 3: Making Predictions with a Model
The relationship between the temperature (T) in degrees Fahrenheit and the number of ice cream cones (N) sold at a shop is modeled by: .
Question: Predict how many ice cream cones will be sold when the temperature is 85°F.
Solution:
Substitute T = 85 into the model:
The model predicts that 620 ice cream cones will be sold when the temperature is 85°F.
Example 4
Example 4: Calculating and Interpreting Residuals
A model for predicting a car's fuel efficiency (E) in mpg based on its weight (W) in thousands of pounds is: . A car weighing 3,000 pounds has an actual fuel efficiency of 28 mpg.
Question: Calculate and interpret the residual for this car.
Solution:
First, convert the weight to thousands of pounds: 3,000 pounds = 3 thousand pounds.
Predicted efficiency: mpg
Residual = Actual - Predicted = 28 - 25 = 3 mpg
The positive residual of 3 mpg indicates that this particular car has a fuel efficiency 3 mpg higher than what the model predicts for cars of its weight. This car is more fuel-efficient than expected.
Example 5
Example 5: Finding a Value Using a Model
The relationship between study time (t) in hours and test score (s) is given by: .
Question: How many hours should a student study to achieve a score of 82?
Solution:
Substitute s = 82 into the model and solve for t:
The student should study for 7 hours to achieve a score of 82 according to the model.
Example 6
Example 6: Identifying Outliers in a Scatterplot
A scatterplot shows the relationship between years of experience (x) and annual salary (y) for employees at a company. Most points follow a clear positive linear trend, but one point representing an employee with 2 years of experience and a salary of $120,000 is far above the pattern.
Question: How would this outlier affect the linear model if included in the calculation?
Solution:
This outlier would pull the regression line upward, especially on the left side of the graph. This would:
- Increase the y-intercept (making the predicted starting salary higher)
- Decrease the slope (making the predicted salary growth per year of experience less steep)
- Make the model less representative of the typical relationship between experience and salary
- Reduce the model's predictive accuracy for most employees
In this case, the outlier might represent a special case (perhaps someone with rare skills or connections) and might reasonably be excluded from the model or investigated separately.
Free Full Length SAT Tests withOfficial-StyleQuestions
Practice with our full length adapive full testreal test-likequestions and proven300+points score boost
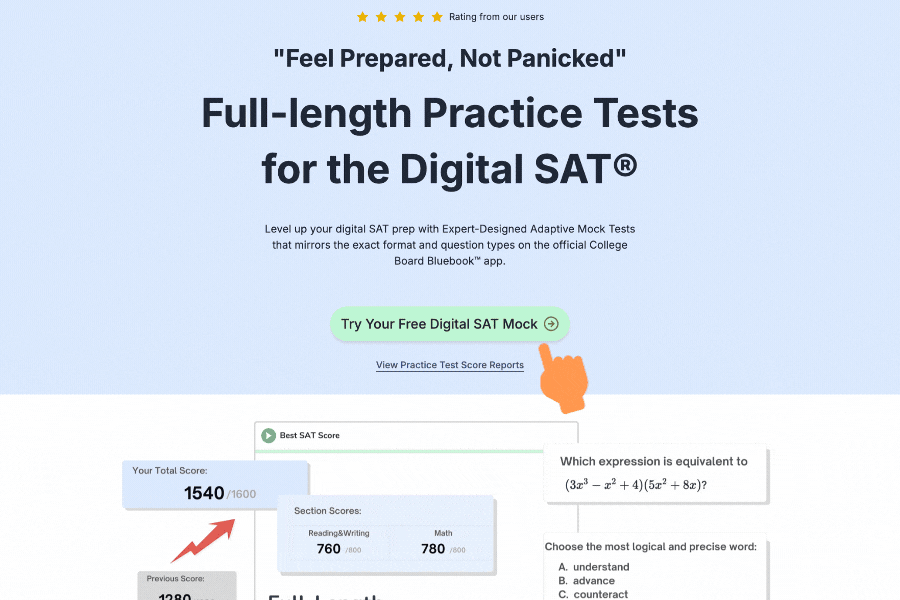
Common Misconceptions
Misconception 1: Correlation implies causation
Reality: A correlation between two variables doesn't necessarily mean one causes the other. For example, ice cream sales and drowning deaths both increase in summer, but ice cream doesn't cause drownings—hot weather influences both.
Misconception 2: A linear model is always appropriate
Reality: Not all relationships between variables are linear. Sometimes data follows curved patterns that require non-linear models. The SAT primarily focuses on linear relationships, but it's important to recognize when a linear model might not be appropriate.
Misconception 3: Extrapolation is always reliable
Reality: Using a model to predict values far outside the range of the original data (extrapolation) can lead to unreliable results. Relationships between variables might change beyond the observed range.
Misconception 4: The line of best fit passes through all data points
Reality: The line of best fit rarely passes through all data points. It's designed to minimize the overall distance between the line and all points, not to connect them.
Misconception 5: A perfect model should have zero residuals
Reality: In real-world data, perfect models are extremely rare. Even good models typically have non-zero residuals for most data points.
Misconception 6: Stronger correlation means causation is more likely
Reality: The strength of correlation doesn't indicate whether a causal relationship exists. A strong correlation might still be coincidental or influenced by a third variable.
Practice Questions for Two-variable data: models and scatterplots
Question 1
Question 1:
A researcher collected data on the number of hours students spent preparing for an exam (x) and their exam scores out of 100 (y). The linear model that best fits the data is .
(a) What is the predicted score for a student who studies for 10 hours?
(b) What does the slope of 2.5 represent in this context?
(c) What does the y-intercept of 60 represent in this context?
(d) A student studied for 8 hours and received a score of 85. Calculate and interpret the residual.
Answers:
(a) . The predicted score is 85.
(b) The slope of 2.5 means that for each additional hour of study time, the exam score is expected to increase by 2.5 points.
(c) The y-intercept of 60 represents the predicted exam score for a student who spends 0 hours preparing for the exam.
(d) Predicted score:
Residual = Actual score - Predicted score = 85 - 80 = 5
The residual of 5 means this student scored 5 points higher than the model predicted for 8 hours of study time.
Question 2
Question 2:
The scatterplot below shows the relationship between the age of a car (in years) and its value (in thousands of dollars) for 12 cars of the same model.
[Imagine a scatterplot showing a negative correlation with points roughly following a downward trend from (0,30) to (10,5)]
(a) Describe the correlation shown in the scatterplot.
(b) The linear model for this data is , where V is the value in thousands of dollars and A is the age in years. Interpret the meaning of the slope in this context.
(c) According to the model, what is the predicted value of a 6-year-old car of this model?
(d) If you wanted to buy a car of this model for $10,000, approximately how old would you expect the car to be according to the model?
Answers:
(a) The scatterplot shows a strong negative correlation between a car's age and its value. As cars get older, their value consistently decreases.
(b) The slope of -2.5 means that for each additional year of age, the car's value is expected to decrease by $2,500.
(c) . The predicted value is $15,000.
(d) We need to find A when V = 10:
According to the model, a car worth $10,000 would be approximately 8 years old.
Two-variable data: models and scatterplots Questions
Question 1
Question 1:
A coffee shop tracked the outdoor temperature (in degrees Fahrenheit) and the number of hot coffees sold each day for two weeks. The data is modeled by the equation , where C is the number of hot coffees sold and T is the temperature.
(a) What does the slope of -5 tell you about the relationship between temperature and coffee sales?
(b) What is the predicted number of hot coffees sold on a day when the temperature is 65°F?
(c) On a day when the temperature was 50°F, the shop sold 330 hot coffees. Calculate and interpret the residual.
(d) According to the model, at what temperature would the shop expect to sell 250 hot coffees?
Answers:
(a) The slope of -5 indicates that for each one-degree increase in temperature, the number of hot coffees sold decreases by 5 cups. This makes intuitive sense as people tend to buy fewer hot drinks on warmer days.
(b) . The model predicts 75 hot coffees will be sold when the temperature is 65°F.
(c) Predicted sales:
Residual = Actual - Predicted = 330 - 150 = 180
The residual of 180 means the shop sold 180 more hot coffees than the model predicted for a 50°F day. This large residual suggests either an unusual day (perhaps it was rainy or there was a special event) or that the model may not be very accurate.
(d)
According to the model, the shop would expect to sell 250 hot coffees when the temperature is 30°F.
Question 2
Question 2:
A real estate analyst collected data on houses in a neighborhood, comparing the size (in square feet) and the selling price (in thousands of dollars). The scatterplot shows a positive correlation, and the linear model is , where P is the price in thousands of dollars and S is the size in square feet.
(a) What does the y-intercept of 50 represent in this context? Is this value likely to be meaningful? Why or why not?
(b) According to the model, how much additional value does each square foot add to a house's price?
(c) If a 2,000-square-foot house in this neighborhood sold for $400,000, how does this compare to the model's prediction?
(d) The analyst wants to predict the selling price of a 5,000-square-foot luxury home in this neighborhood using the model. Explain one reason why this prediction might not be reliable.
Answers:
(a) The y-intercept of 50 represents the predicted price ($50,000) of a house with 0 square feet, which is not meaningful in reality since a house cannot have 0 square feet. This illustrates that the y-intercept sometimes lacks practical interpretation, especially when x = 0 is outside the realistic domain.
(b) The slope of 0.15 means each additional square foot adds 150).
(c) Model prediction for a 2,000-square-foot house: thousand dollars, or 400,000
The house sold for $50,000 more than the model predicted, suggesting it may have had premium features, a desirable location within the neighborhood, or other factors that increased its value beyond just its size.
(d) The prediction might not be reliable because:
- The 5,000-square-foot home may be outside the range of the original data used to create the model (extrapolation).
- Luxury homes often have premium features that add value beyond just square footage.
- The relationship between size and price might not remain linear for very large homes.
- The market for luxury homes might behave differently than the market for average-sized homes in the neighborhood.
Two-variable data: models and scatterplots Learning Checklist
I can identify the independent and dependent variables in a two-variable relationship.
I can interpret a scatterplot to determine the direction (positive, negative, or no correlation) and strength of a relationship between variables.
I can interpret the slope of a linear model in context, explaining what the rate of change means for the specific variables involved.
I can interpret the y-intercept of a linear model in context, recognizing when it has practical meaning and when it doesn't.
I can use a linear model to make predictions by substituting values into the equation.
I can calculate and interpret residuals to assess how well a model fits specific data points.
I understand the limitations of linear models, including the dangers of extrapolation and the fact that correlation does not imply causation.
I can solve for unknown values in a linear model when given other information.
I can identify outliers in a scatterplot and understand how they might affect a linear model.
I can apply two-variable data analysis skills to real-world contexts and SAT problems.
Essential SAT Prep Tools
Maximize your SAT preparation with our comprehensive suite of tools designed to enhance your study experience and track your progress effectively.
Personalized Study Planner
Get a customized study schedule based on your target score, available study time, and test date.
Expert-Curated Question Bank
Access 2000+ handpicked SAT questions with detailed explanations, organized by topic and difficulty level.
Smart Flashcards
Create and study with AI-powered flashcards featuring spaced repetition for optimal retention.
Score Calculator
Convert raw scores to scaled scores instantly and track your progress towards your target score.
SAT Skills Lessons
Master each SAT skill with progressive lessons and comprehensive guides, from foundational concepts to advanced techniques.
Full-Length Practice Tests
Experience complete SAT exams under realistic conditions with adaptive difficulty.
Pro Tip
Start your SAT prep journey by creating a personalized study plan 3-4 months before your test date. Use our time management tools to master pacing, combine mini-tests for targeted practice, and gradually progress to full-length practice tests. Regular review with flashcards and consistent practice with our question bank will help you stay on track with your study goals.